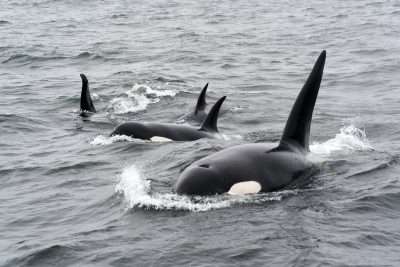
Photo by Mike Doherty on Unsplash
Scientists turned to the next best thing: bio-logging devices that can be attached to animals and capture information about movement, breathing rate, heart rate, and more.
However, retrieving an accurate picture of what a tagged animal does as it journeys through its environment requires statistical analysis, especially when it comes to animal movement, and the methods statisticians use are always evolving to make full use of the large and complex data sets that are available.
A recent study by researchers at the Institute for the Oceans and Fisheries (IOF) and the UBC department of statistics has taken us a step closer to understanding the behaviours of northern resident killer whales by improving statistical tools useful for identifying animal behaviours that can’t be observed directly.
“The thing we really tackled with this paper was trying to get at some of those fine-scale behaviours that aren’t that easy to model,” said Evan Sidrow, a doctoral student in the department of statistics and the study’s lead author. “It’s a matter of finding behaviours on the order of seconds—maybe 10 to 15 seconds. Usually, it’s a matter of a whale looking around, and then actively swimming for a second to get over to a new location. We are trying to observe fleeting behaviours, like a whale catching a fish.”
The research team improved a statistical tool that is based on what is called a hidden Markov model, which is helpful for unlocking the mysteries hidden inside animal movement datasets.
“Traditional hidden Markov models break down at very fine scales,” Sidrow said. “That’s because there’s structure in the data you can’t capture using the basic type of hidden Markov model. We’re trying to capture it with this model—we’re trying to account for this ‘wigglyness’ that a traditional hidden Markov model wouldn’t be able to account for.”
In other words, now that tags can collect data almost continuously, researchers are left with an immense number of data points taken fractions of seconds apart, and traditional Markov models and statistical methods struggle to interpret such high-frequency information—hence the need for the more advanced Markov model proposed in the study.
Using the enhanced hidden Markov models, the team found some undiscovered northern resident killer whale behaviours. The whale they used to develop the model preferred to save energy by gliding through the water when making deep dives, and when it was closer to the surface, it moved more actively, accelerating faster and ‘fluking’ its tail more often.
Understanding these diving patterns will be crucial for whale conservation because it will help researchers learn how much energy the whales require to sustain themselves.
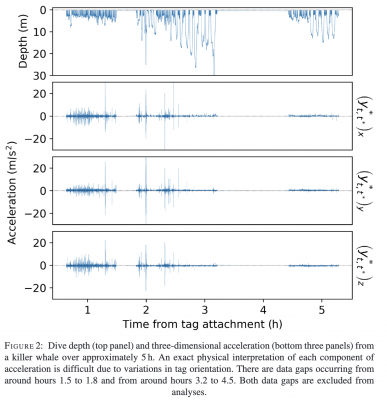
Figure 2 – Sidrow
“It could be applied to pretty much any animal movement data,” he said. “If you’re tagging animals and you want to understand fine-scale behaviours, then this method that could be useful—even for things like the flapping of birds’ wings.”
It could even prove useful in areas outside of ecology, such as determining when machines are likely to break by classifying when the parts inside of them are vibrating abnormally.
The work is one of the first steps on the road to fully understanding why southern resident killer whales are not faring as well as their northern counterparts, according to Dr. Marie Auger-Méthé, senior author of the study and an assistant professor in the department of statistics and the Institute for the Oceans and Fisheries.
“Using our methods to detect when the animals are catching prey and to model their energy expenditure will be key to understanding the differences between these neighbouring whale populations,” she said.
The next goal is to understand when the whales are capturing prey and applying the models to both northern and southern resident killer whale populations to see how they are behaving differently.
“The paper offers many ‘building block’ solutions that can be used together or independently,” Dr. Auger-Méthé said. “In essence, we are providing a toolbox to researchers using high-frequency movement data, and other similar high-frequency time series.”
“Modelling multi-scale, state-switching functional data with hidden Markov models” was published in The Canadian Journal of Statistics.
Tags: Animal movement, killer whales, Marie Auger-Methe, statistical ecology, whales